TECH
Generative AI Development: Trends and Challenges in Emerging Tech
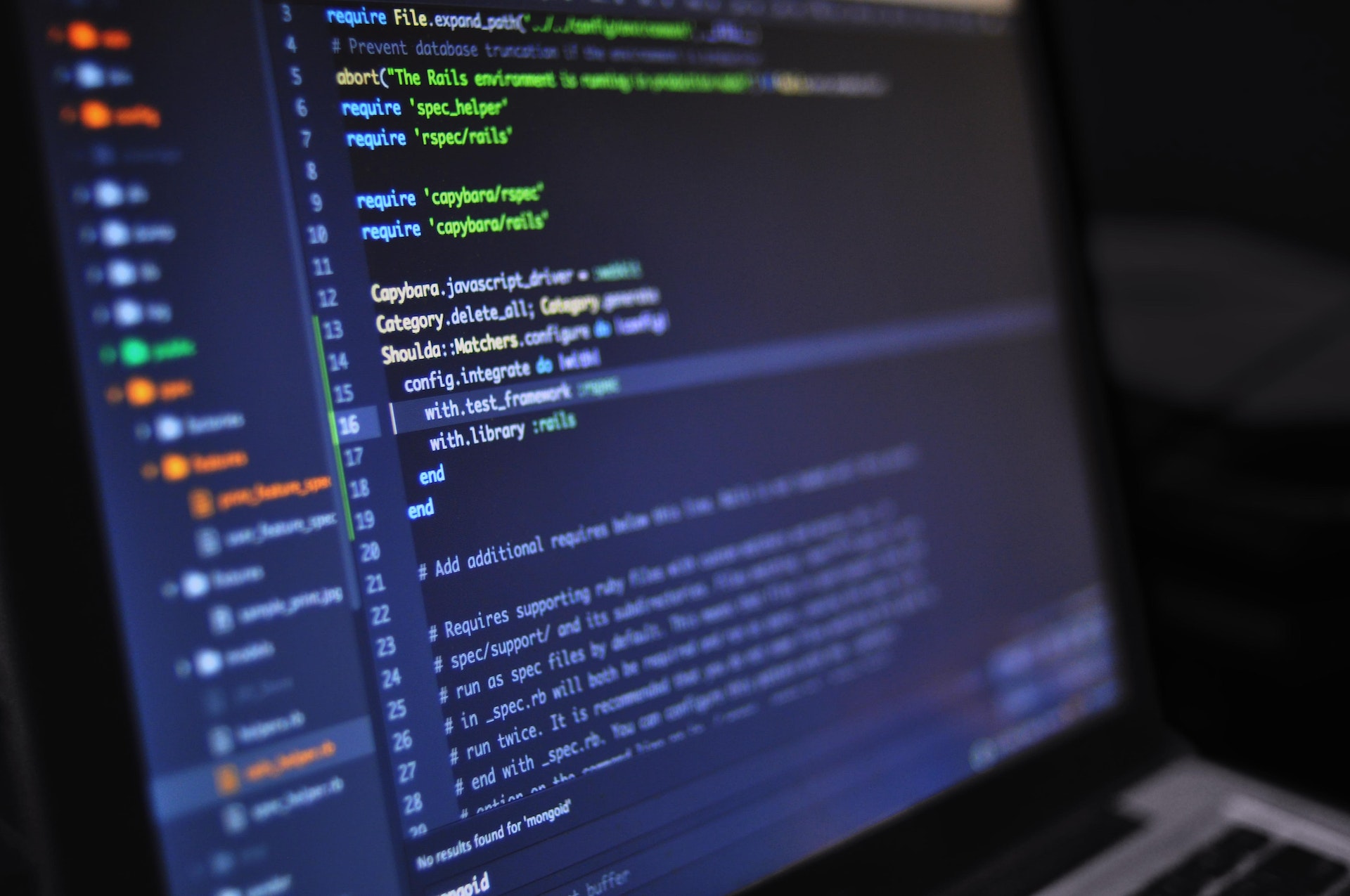
Generative AI represents a paradigm shift in artificial intelligence, where the focus is on creating new content rather than just analyzing existing data. Through a variety of models and tools, it enables the generation of creative content, such as text, audio, images, and even code. The technology is poised for significant growth, with predictions that by 2025 a notable portion of enterprises will have adopted AI-augmented development and testing strategies.
Central to the development of Generative AI is a progressive enhancement in model quality and user control. This evolution mirrors the rising demand for customizability and multimodal applications, which can cater to diverse and complex requirements. Innovations are continuously emerging in areas like few-shot and one-shot learning, highlighting the technology’s improvement in learning and applying knowledge with minimal data input.
Generative AI is not merely about generating content but also about its potential economic impact. Early adopters of the technology may see measurable growth in their operational efficiency and outputs. This transformative capability of Generative AI is anticipated to contribute marginally to global economic growth, especially for those who integrate and leverage these systems early on in their developmental strategy.
Fundamentals of Generative AI
Generative AI represents a shift in how machines understand and create content, focusing on the generation of new, original material from existing data.
Understanding Generative Models
Generative models are at the heart of Generative AI, designed to learn patterns and distributions within input data. They can synthesize data that is similar to what they have been trained on, creating everything from text to images. By sampling from the learned data distributions, these models can produce novel content or make predictions for incomplete data sets.
Types of Generative AI
Generative AI encompasses a variety of model types, each ideal for different applications:
- Probabilistic Models: They estimate the probability of outcomes, useful for predicting future events or completing incomplete data.
- Autoregressive Models: Using a sequence of past data, they predict the next output iteratively.
- Generative Adversarial Networks (GANs): Comprise a generator and a discriminator, working in tandem to produce increasingly accurate outputs.
- Variational Autoencoders (VAEs): Employ a probabilistic spin on the traditional autoencoder, generating new instances that are likely under the model’s learned distribution.
Key Algorithms and Architectures
Generative AI relies on sophisticated algorithms and architectures to function effectively:
- Deep Learning Neural Networks: Offer the backbone for many generative models, learning complex patterns in large datasets.
- Recurrent Neural Networks (RNNs): Excel in processing sequential data, fundamental for models that generate text or other data that follow a sequence.
- Transformer Architectures: Newer models that have demonstrated significant capabilities, especially in natural language processing.
Through these technologies, Generative AI continues to evolve, offering innovative solutions across various fields and applications.
Read also: Custom AI Software Development: Streamlining Business Processes
Application and Implications
Generative AI is reshaping numerous industries with its ability to create novel content and streamline processes. This section explores its diverse applications, scrutinizes the ethical considerations it raises, and anticipates its future developments.
Practical Applications
Generative AI finds its use in diverse domains, from content creation to complex problem-solving.
- Content Generation: It excels in creating unique text, images, videos, and music that can aid in marketing, education, and entertainment.
- Design and Engineering: Industries like automotive and architecture benefit from AI’s capability to visualize new product designs and optimize structures through simulation.
- Healthcare: By generating synthetic data, generative AI assists in medical research, facilitating drug discovery and tailored treatment plans.
Ethical Considerations
The rise of generative AI also poses significant ethical challenges.
- Data Privacy: As these systems often train on vast amounts of data, ensuring that this data does not compromise user privacy is critical.
- Bias and Fairness: AI systems can unconsciously perpetuate biases present in their training data, necessitating rigorous audits for fairness.
- Authenticity: The ability of Generative AI to create convincing fake content necessitates robust verification mechanisms to maintain trust and transparency.
Future Prospects
Generative AI is poised for significant growth and sophistication.
- Advancements in Technology: Ongoing research promises more advanced models that produce higher fidelity outputs with less input.
- Integration Across Sectors: One can anticipate its integration into more sectors, streamlining operations and fostering innovation.
- Regulatory Evolution: As the technology matures, so too will the legal frameworks that guide its ethical and responsible use.
The article was written in cooperation with the experts of SoftKraft – Generative AI Development Services
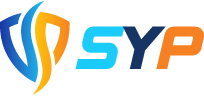
-
ENTERTAINMENT1 month ago
Cinemark: Elevating the Movie-Going Experience
-
EDUCATION1 month ago
LET Result October 2022: Full List of Passers and Topnotchers
-
ENTERTAINMENT1 month ago
JJK Chapter 248: Major Plot Twists and Intense Battles Unfold
-
GAMES1 month ago
Pokemon Emerald Rare Candy Cheat: How to Maximize Your Team’s Potential